Predictive CLV: how to optimize your ROI
Time to stop looking into your crystal ball – the predictive customer lifetime value (CLV) lets you really get to know your customers and what they want. But how can you effectively leverage your data? And how does a CLV prediction work?
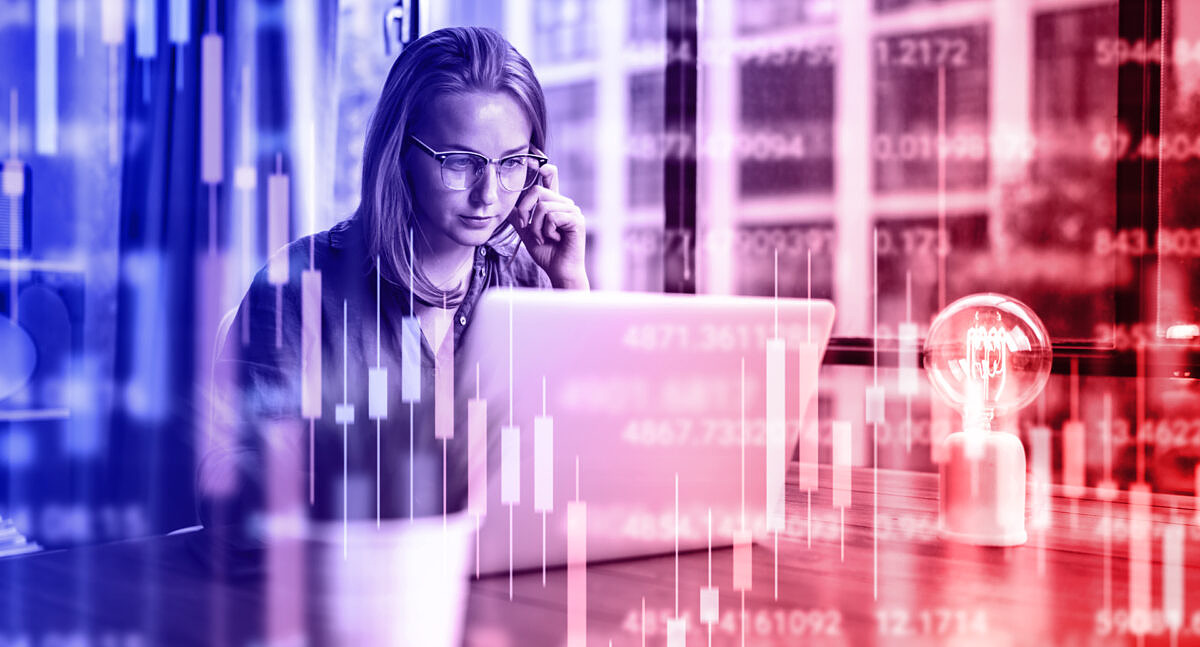
Know your customers: why the predictive CLV is a must
Most of us have a favorite restaurant that has been our home away from home for years – somewhere we go at least once a week with friends or family to eat, drink, and enjoy ourselves. As a regular customer, we’re greeted here by name, are given personalized recommendations, and have our every need catered to.
The costs that our go-to establishments invest in us are negligible: every now and again we’ll get a dessert on the house or our table will be treated to a complimentary round of drinks. But what really makes us go back time and time again? The answer is probably: human connection. And our favorite restaurants achieve that because they know us inside out and can give us exactly what we want.
E-commerce: powerful data for a precise CLV
When it comes to products or services, though, it’s often a lot harder to figure out what your customers like and what matters to them.
The solution: the predictive customer lifetime value, or CLV for short. E-commerce offers great scope for capturing ample data that you can use to create highly precise CLV models for your customers.
Customer lifetime value analysis: how do you calculate the predictive CLV?
To calculate the CLV of a customer, you need to perform a customer lifetime value analysis. There are three ways of doing that:
#1 The average customer lifetime value across all your customers
This method only really scratches the surface and merely gives you an overview of the average customer.
A specific time period is defined, for example the last year. At the start of this period, your favorite restaurant had 250 customers. By the end of the period, it had attracted 100 new customers. On the other hand, now, there are a total of 300 customers in the customer base. On average, each customer visited the restaurant once a month (equating to a purchase frequency rate of 12) and spent 30 dollars each time.
First, the average customer lifespan is calculated. To do that, the 300 total customers are subtracted by the new customers, and this number is then divided by the number of existing customers before the time period under consideration: (300 – 100) / 250 = 0.8. This value is the customer retention rate, namely 80 percent. This means that 80 percent of your customers have stayed with you over the one-year period, while 20 percent have left. If you then calculate 1 / (1 – 0.8), you get the customer lifespan in years – which is 5 in this case.
The total number of customers (300) is then multiplied by the order value (30 dollars) and the purchase frequency rate (12). In this example, that amounts to 108,000 dollars. When multiplied by the customer lifespan calculated earlier, this results in a customer lifetime value of 540,000 dollars for the next 5 years. Per person, that equates to 1,800 dollars.
#2 The individual predictive customer lifetime value
This value is much more precise, but you need the right data in order to calculate it. Otherwise, the calculation is very similar. Using the same example as before, the formula is as follows: average order value (30 dollars) multiplied by the purchase frequency rate (50 per year) and the customer lifespan (10 years). That reveals that the customer will spend 15,000 dollars over the next 10 years.
And this is when it gets interesting: your colleague also likes your go-to restaurant. In contrast, they only eat there once a year but pay the bill for the whole team. This would be calculated as follows: 4,000 dollars (order value) x 1 (purchase frequency rate) x 8 (customer lifespan) = 32,000 dollars.
So although your colleague only visits the restaurant once a year, they’re a valuable customer, at least in terms of order value. And that’s where the strength of the individual predictive CLV lies: it allows you to make extremely precise predictions and determine which customers are worth investing in.
#3 Predictive customer lifetime value using machine learning
Of course, even better data can lead to a much more precise calculation. Measuring the predictive CLV using machine learning is all about algorithms. These can be ascribed to customers or other values and then aggregated. Using well-known parameters such as click behavior, time on site, and return rate, accurate predictions can be made about each customer.
Another advantage is that the algorithms continue to learn every day by comparing historical and current data, identifying trends, and using those insights to recalculate the CLV on an ongoing basis.
Predictive CLV: math that pays off!
Customer acquisition is one of the most important factors for business growth, but it requires time, money, and resources. New customers matter, but returning customers matter even more. The example of a favorite restaurant highlights how companies and businesses can benefit from investing in existing customers – because not only will they return, they’ll also bring new customers with them!
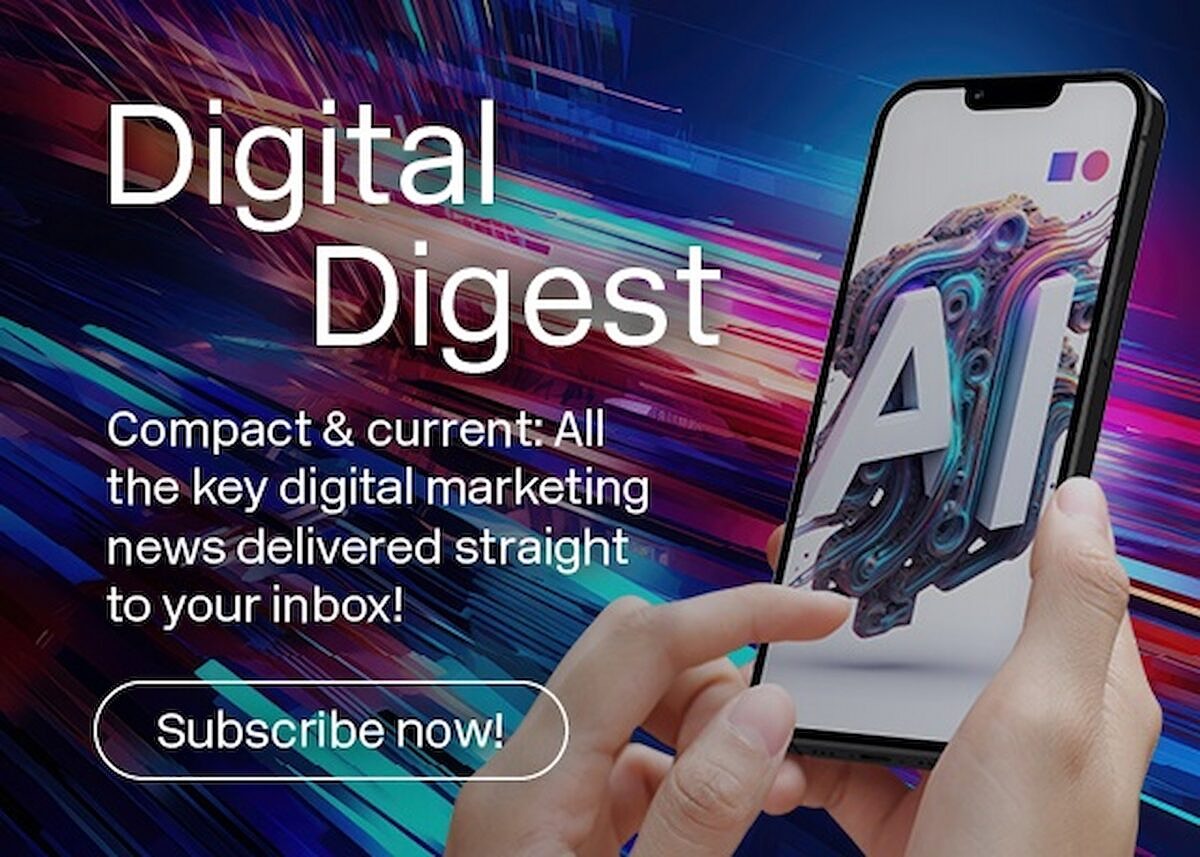