Successful A/B testing in e-commerce: Why precise hypotheses are important
A/B testing is an efficient instrument to let shop owners optimize their websites. Precise hypotheses lay the foundation for meaningful results. Our free template shows you how to define them to achieve a constructive outcome.
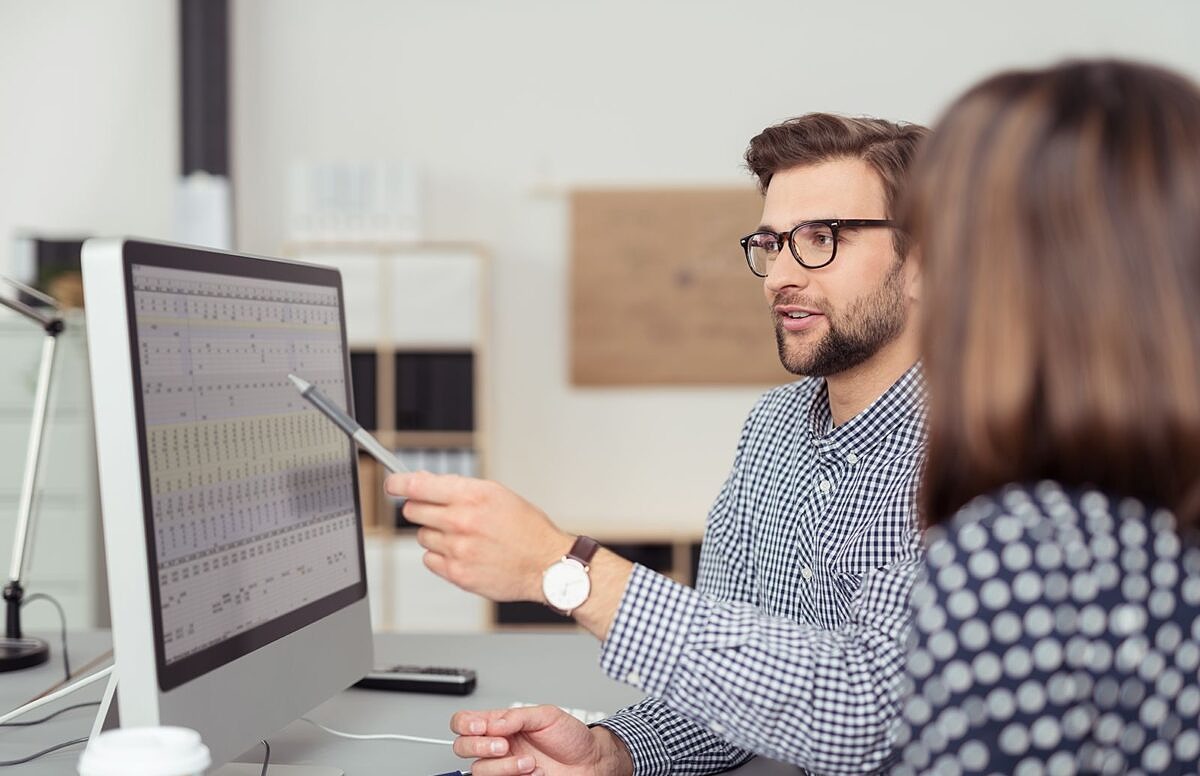
A/B testing for a better customer experience
Website performance plays a decisive role in a business’s success, especially where online shops are concerned. Even miniscule changes can have a huge impact on user behavior and thus on conversion rates. If, for example, a simple change to an online form impairs the usability of the checkout process, the number of aborted purchases will rise.
Continuous A/B testing in e-commerce helps avoid such problems in the customer experience. When skillfully set up, they elucidate how changes to pages, advertisements or e-mails can affects customer behavior – and ensure that customers conclude their transactions faster.
Popular tool for marketers
A/B testing, as a data-driven process, is one of the most widespread methods for testing different messages and deriving concrete recommendations for action. From a technical perspective, A/B testing is far less complex than multivariate analyses. It is also very fast to deliver important findings, especially in concrete applications with few variations of a key element. Precisely this simplicity, combined with the growing range of data analytics possibilities, makes A/B testing a popular tool for online shops.
Numerous use cases for A/B testing
The range of use cases in which A/B testing is regularly employed is correspondingly broad, and includes:
- Homepage (e.g. CTA clicks)
- Category page (e.g. length of stay)
- Product page (e.g. conversions)
- Landing page (e.g. bounce rates)
- E-mail (e.g. read rates)
- SEO (e.g. organic traffic)
- Ads (e.g. impressions)
Whether for landing page optimization or checkout tweaking – the tests should be carried out in various segments to maximize their meaningfulness. The reason for this is that user behavior and their expectations of how content is presented differ depending on the devices and channels (e.g. mobile or desktop) employed.
Define the goals of A/B tests
A precise definition of the objectives is essential for efficient use of A/B testing. These determine where, and on which key elements, work is possible. Where the focus is on bounce rates, for example, tweaking information density or rearranging certain elements could offer good starting points for comparative tests.
Formation of hypotheses as the starting point for A/B testing
Objectives for the planned A/B tests should not be defined on the basis of gut feeling or subjective impressions, but by forming sound hypotheses. Consequently, the starting point for any analysis should always be a precise hypothesis that allows the best possible evaluation of the test findings later on. A/B testing can thus be broken down into four steps:
- Hypothesis definition
- A/B test planning (including random sample size, duration, participants, influencing factors, significance)
- Data capture
- Data evaluation
Hypothesis quality is decisive for the success of A/B tests
Not every assumption is suitable as a hypothesis for A/B testing. Vague formulations such as “The redesign of landing page X leads to a tangible improvement in conversion rates” lack the necessary conceptual depth, and cannot be validated. The success of A/B tests therefore depends largely on the quality of the initially formulated assumptions.
Precisely formulated hypotheses establish a measurable connection between an observed problem, the planned change (key elements) and the expected change in user behavior. The four elements of a well thought-out hypothesis are derived from these requirements:
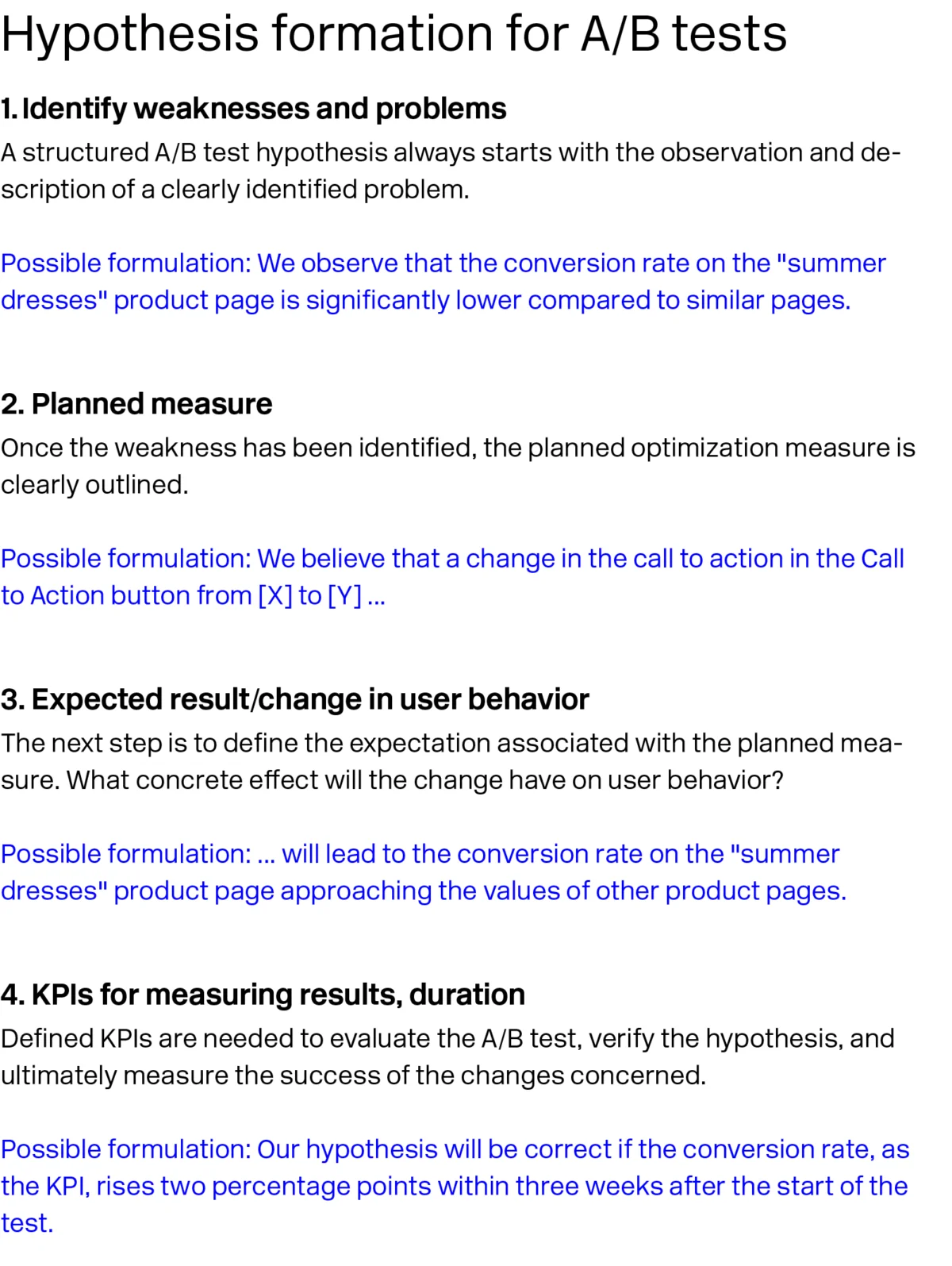
Free hypothesis template for A/B tests as an orientation aid
Well-structured, precisely formulated hypotheses are among the essential success factors in A/B testing. Our free template offers you a good overview of the four elements for formulating hypotheses and gives you practical help in doing so.