DMEXCO marketing precap part 3: data trends of 2021
In the medium term, long-established data collection strategies will no longer bring the desired success. Marco Szeidenleder, founder and Managing Partner of Pandata, shows us what data trends to expect in 2021.
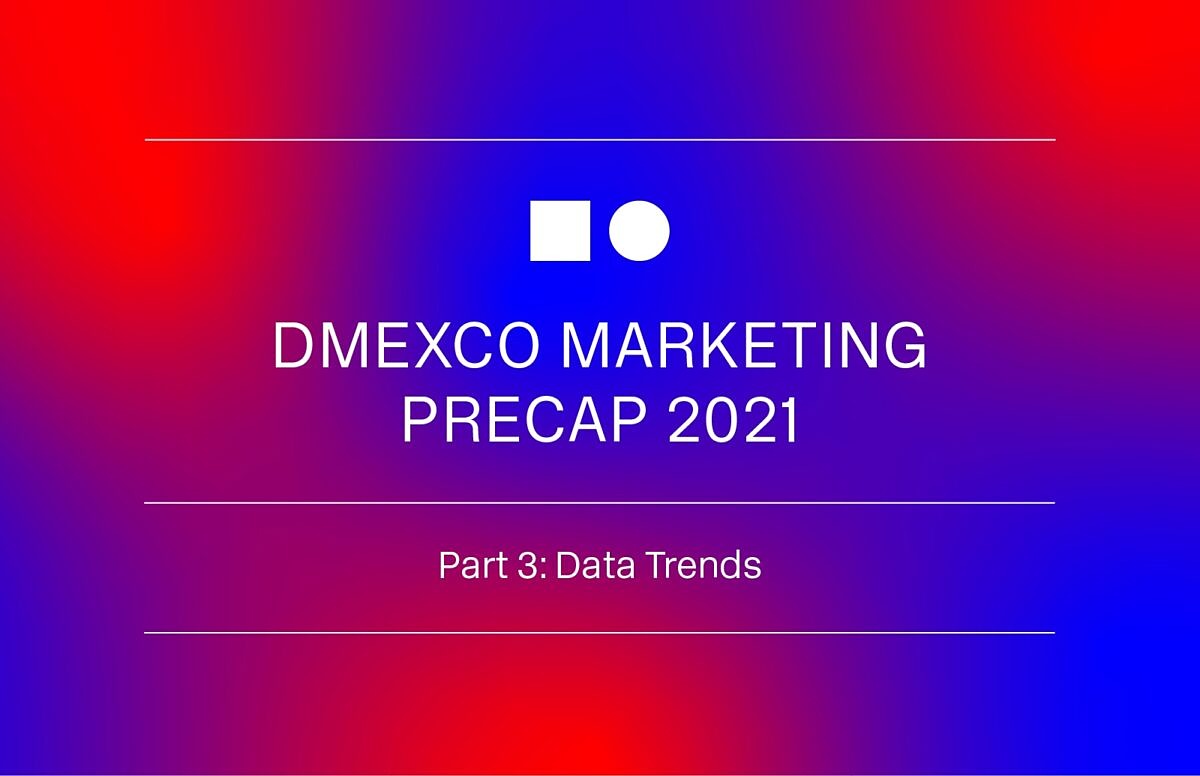
Analyzing data for marketing purposes is being made more and more difficult because individuals are generally becoming increasingly concerned about their data privacy – something that is technically and legally shaking up how data is collected and used. Consequently, a large number of website users are now resorting to ad blockers in order to effectively prevent data from being collected by means of conventional methods. Some browsers or browser plug-ins are even going as far as deliberately sending false data to marketing tools.
Browser manufacturers are adding fuel to the fire and positioning themselves on the market by offering packages of protection mechanisms to stop user data from being collected. The Mozilla Firefox “Enhanced Tracking Protection” (ETP) and Apple Safari’s “Intelligent Tracking Protection” (ITP) are leading the way here, and even major platforms are increasingly taking a stance against the use of data. Accordingly, Facebook stripped down the functionality of its API some time ago, and Apple also introduced new device ID restrictions for iOS that make it harder for advertisers to identify users.
European legislation now not only requires users to actively consent to virtually any kind of data collection, but it is also posing a significant obstacle to using solutions from outside the EU. In particular, the fact that the USA has been deemed an “unsafe” country when it comes to data privacy has led to the most common tools, including Google Analytics, becoming a legally risky gray area.
Status quo
In recent years, cookies have become synonymous with the illegitimate use of data. They form the backbone of Internet technology, and although there are certainly other ways to store data in a browser, they are practically the only method that makes it possible to identify users across websites – and thus enabling ads to “track” users. There are still some workarounds attempting to maintain the status quo, for example technical tricks for extending cookie duration or other identification techniques like fingerprinting.
With the latter, a unique ID is generated from the user’s browser data for identification purposes. These workarounds are still able to function effectively, but sooner or later they’ll be prevented by browser manufacturers. Alongside technical mechanisms, dark patterns, i.e. designing a website in such a way that users are tricked into consenting to their data being collected, will also soon be perceived as digital sleights of hand and consequently disappear.
User data is currently still collected almost exclusively in the website user’s browser. This approach previously offered a range of advantages, such as unrestricted access to cookies and easy implementation of tracking codes. Now though, the aforementioned points make this client-side tracking more difficult and less thorough, mainly because collecting data via a browser is completely controlled by how that browser handles data. Some of the problems associated with this can be solved by switching to server-side data collection.
Server-side tracking
Even though server-side tracking is as old as web analytics itself, recent developments are enormously boosting its relevance and it is expected to be one of the big marketing buzzwords of 2021. It works as follows: When a user visits a website, it is called up from a web server. The web server therefore always “knows” about the user’s behavior on the website to a certain degree. Server-side tracking utilizes this unavoidable connection, since the data is sent directly to the analytical tools from the web server rather than from the user’s browser (i.e. the client).
The browser has nothing to do with the data collection, meaning that the whole process is completely hidden from the user. In this way, server-side tracking is not hindered by ad blockers or even browser mechanisms like ETP and ITP, thereby delivering almost flawless data quality. Since there is no point of contact with the browser during tracking, not only do the page load times remain unaffected, but it is also possible to enrich tracking data during collection.
As a result, critical data, such as product margins, can be incorporated in real time into the tracking and also into the communication with marketing channels.
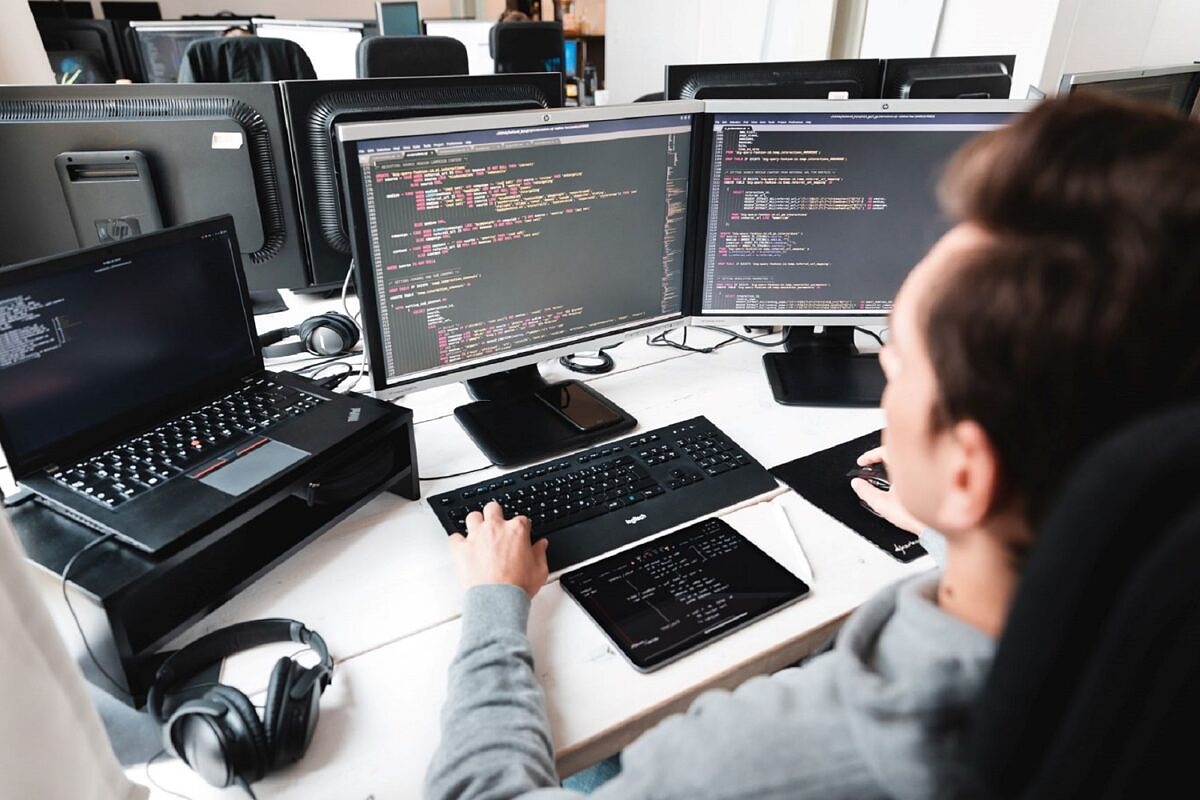
The approach’s much more complex implementation and limited possibilities for accessing cookies have so far stopped it from becoming more prevalent. There is now a vast array of tools to at least significantly simplify implementation, while cookies will be considerably less relevant in the near future.
Server-side tagging
Some tracking tool providers have long integrated server-side tracking as part of their products. Although the Google solution is still relatively new to the market, Google’s market power and the innovative hybrid approach give reason to believe that it will really take off in the future. Server-side tagging essentially combines server-side and client-side tracking, and although it still involves data being collected in the browser in the traditional way, that data is not sent directly to the analytical tools, but instead directed to a self-hosted instance in the Google Tag Manager for further distribution.
The technology thus enables companies to immediately leverage at least some of the benefits of server-side tracking, but more importantly it gently eases them into the world of server-side tracking. The server-side Google Tag Manager is also capable of receiving tracking data directly from the web server or other sources (CRM system, backend, etc.), which gradually reduces the amount of data collected via the browser.
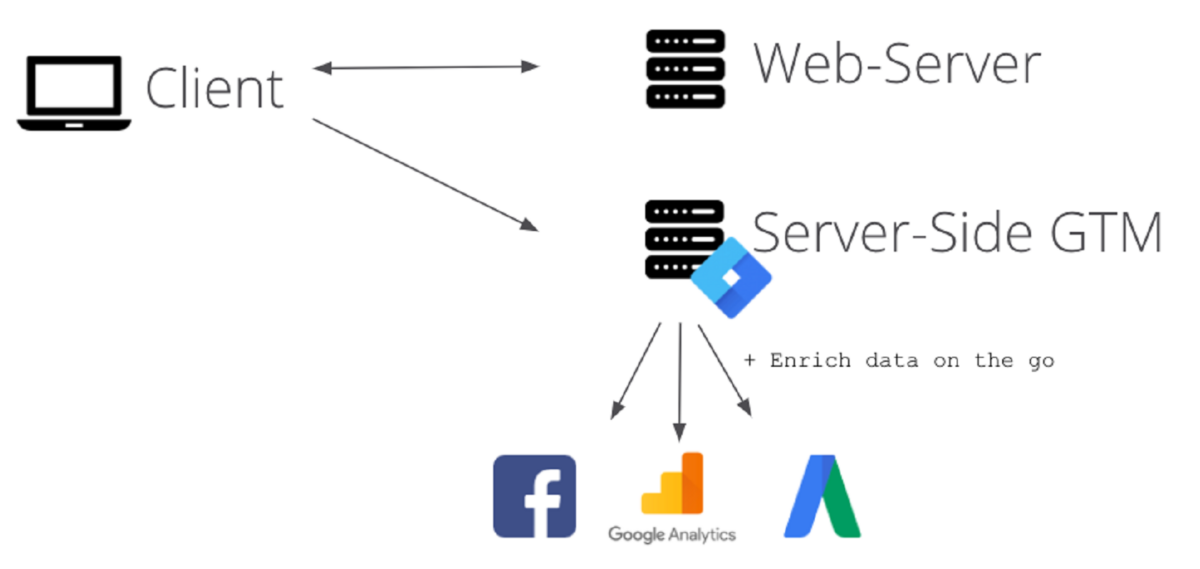
Server-side tracking is not GDPR-exempt: consent will still have to be obtained before data can be collected. Even though more users will probably slip through the net in the future on the whole, the data that will be collected is likely to be of a better quality. Clearly communicated data ethics will play a greater role in all this and will become an integral part of corporate social responsibility. Users will potentially be willing to consent to their data being collected if they understand how it will be used and for what purpose.
Cloud data warehousing
With data collection becoming less browser-based, the technologies and methods used to process data for marketing purposes will also shift their focus to include more business intelligence and data science. Technical systems will additionally need to be networked to a greater extent.
If only to comply with the GDPR, companies must be able to delete a user’s data in its entirety across several systems. It should also be added here that certain use cases only become possible in the first place by merging data from different systems. This process will be driven by a flexible and scalable data warehouse.
When it comes to implementing a flexible data warehouse, cloud systems such as Google BigQuery are now clearly being favored. Other high-performance systems are also available at a much lower price. The purely infrastructural costs of a data warehouse on Google BigQuery, including complex attribution logic, only amount to one or two euros per day, even for a large online retailer.
The increasingly integrative capabilities of the platforms offer added benefits. For example, BigQuery already allows some data sources, such as Google Ads and YouTube, to be tapped into without extensive integration, and the growing popularity of Google Analytics 4 (GA4) now even means that raw data is available without a 360 license.
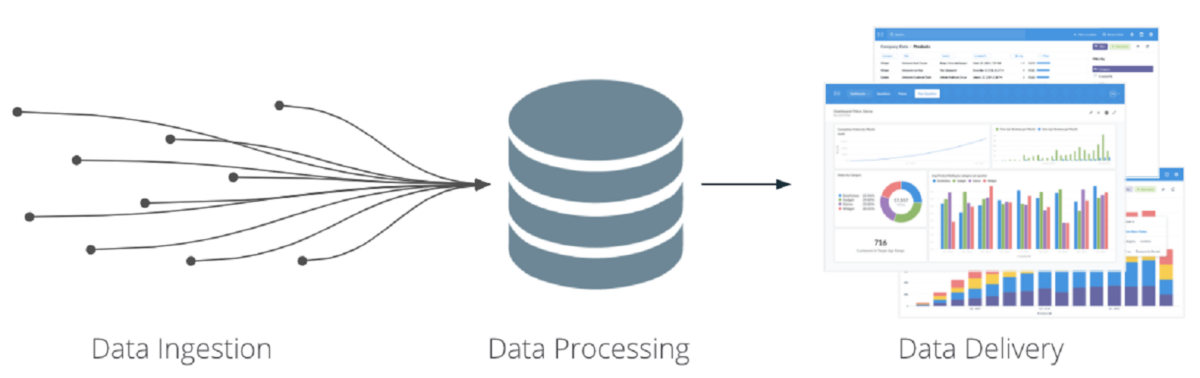
Large-sized companies in particular often have a relatively rigid system architecture that has been in place for many years. That will still do the job for many applications, but frequently won’t measure up to the increased demands of modern data applications in terms of flexibility and scalability. The ability to easily integrate new data sources and the flexibility to also feed third-party systems with data on top of traditional analysis are important aspects.
As the organizational “single source of truth”, the data warehouse also holds the logical definitions that form the basis of subsequent data applications and in this sense prevents multiple versions of the customer lifetime value being used within a company, for example. A modern data warehouse is the solid foundation for data science and machine learning applications.
Data science approaches
Now that the hype surrounding data science has died down, relevant approaches are slowly being put into practice. An example that has already been picked up by the buzzword radar for 2021 is marketing mix modelling, which determines the impact of marketing measures and external factors on a dependent variable, for example sales.
Product and campaign data, marketing expenditure, information on seasonalities, and macroeconomic data provide the output data. In addition to information on the marginal benefit of individual campaigns – “At what point is it no longer worth investing even more money in a campaign?” – the model can algorithmically calculate the effect of different budget allocations on sales and support budget-related decisions.
The bottom line
Far-reaching changes are making it necessary to rethink data collection. In the medium term, some of the familiar and long-established strategies will no longer bring the desired success. In view of this, data collection is shifting from client to server and the backend data infrastructure will become increasingly relevant for marketing. Once clean data collection and a corresponding data infrastructure have been established, it might therefore be wise to use statistical methods to support marketing decisions.