AI agents: Context-based intelligence profiles as a booster for brands
A DMEXCO column by Evgeny Popov on AI agents and the contextual advertising revolution.
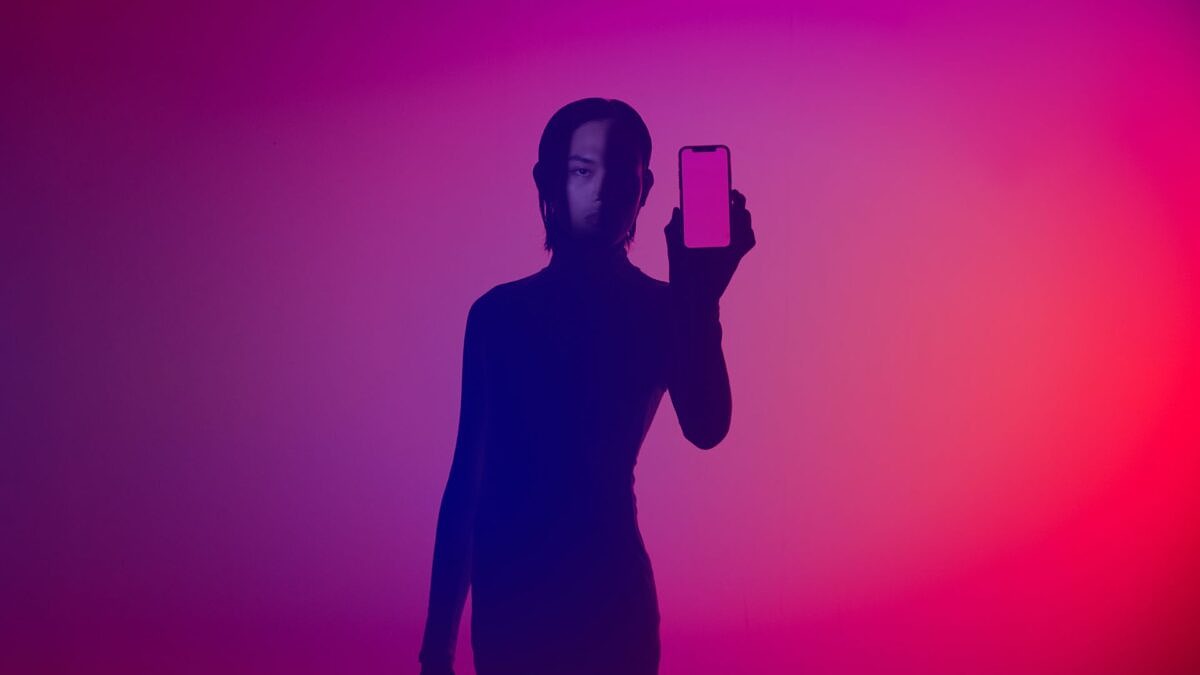
The Future of Context Agents for Smarter Brand Decisions
As marketers demand more granularity, automation, and intelligence in media decision-making, the role of artificial intelligence is rapidly evolving. Traditional NLP-based systems, once seen as groundbreaking, are no longer enough to navigate today’s fragmented, signal-rich, and authentic environments. We are now entering the era of Agentic AI — systems that don’t just understand language, but act on it dynamically, contextually, and strategically.
At the heart of this shift is the convergence of Natural Language Understanding (NLU) with context-based AI agents — a powerful combination that empowers advertisers to move from keyword targeting to real-time, multidimensional media planning. While NLU is a subfield of NLP, has matured into a foundational layer for interpreting user intent, sentiment, and meaning from text. But the next frontier isn’t merely linguistic — it’s agentic.
This article explores how this convergence works, how it can be operationalized using JSON-based outputs, and how it redefines the way we approach suitability, alignment, personalization, and performance in contextual advertising. This synthesis promises a new paradigm in user engagement — where AI systems don’t just parse language, but act on it with agency, personalization, and purpose.
The Limitation of Standalone NLU
NLU systems excel at parsing human language into structured meaning — extracting intent, emotion, topics, and sentiment. In marketing, this powers chatbots, social listening, content moderation, and semantic search. But NLU is often stateless and reactive. It answers questions like:
- What is the topic of this article?
- Is the sentiment positive or negative?
- What product did the user mention?
While useful, these insights lack longitudinal context, goal alignment, or memory. NLU doesn’t determine whether a specific content opportunity aligns with a brand’s messaging, assets, or KPIs — nor does it score risk, engagement, or competitive exposure.
Introducing context-based AI agents: The Missing Layer
Context-based AI agents or Context Agents, as popularized by Bokonads’ The End of Taxonomy, go far beyond NLU by acting as persistent, self-updating intelligence profiles. A Context Agent retains state over time, adapts to goals, learns from feedback, and makes media decisions across multiple vectors.
For advertisers, this means you’re no longer just tagging content for sentiment or relevance — you’re deploying an agent that actively evaluates whether a particular content environment, audience profile, and creative strategy intersect in a way that justifies investment.
A Holistic Evaluation Framework
To operationalize this, we developed a schema that expands the foundational questions marketers typically ask:
- Is this content brand-safe?
- Is this content aligned with our brand identity?
- What message/product should we feature?
- What creative assets should be used here?
But real-world execution demands more than that. Advanced contextual evaluation now includes:
- Who is the audience, and do they match our target?
- How will this content affect brand sentiment?
- Is the content engaging enough for our ad to stand out?
- Does it match our campaign stage (awareness, consideration, conversion)?
- Are there competitive risks here?
- Is the content culturally or temporally relevant?
- Is the environment measurable and attributable?
- Is it compliant with legal/platform standards?
The JSON Schema: Structured Intelligence for Programmatic Decisions
Real-World Example: Brand + Content Scenario
🏷 Brand:
EcoTech Living — a sustainable smart home brand targeting millennial parents.
🎯 Products:
- SmartAir Eco Purifier
- ParentTech Subscription Bundle
📍 Campaign Goal:
Top-of-funnel brand awareness with an Earth Day push.
📺 Content Opportunity:
An article on ParentingGreenLife.com titled:
“How Millennial Parents Are Leading the Sustainability Movement”.
- Positive sentiment
- High engagement across social platforms
- Audience: Urban, sustainability-minded parents
- Cultural hook: Earth Day
- Zero adjacent competitor placements
- Meets GDPR and platform ad policies
System Prompt with Embedded Schema
For teams integrating this into LLMs or proprietary media tools, here’s an example of a system prompt to generate the structured output above:
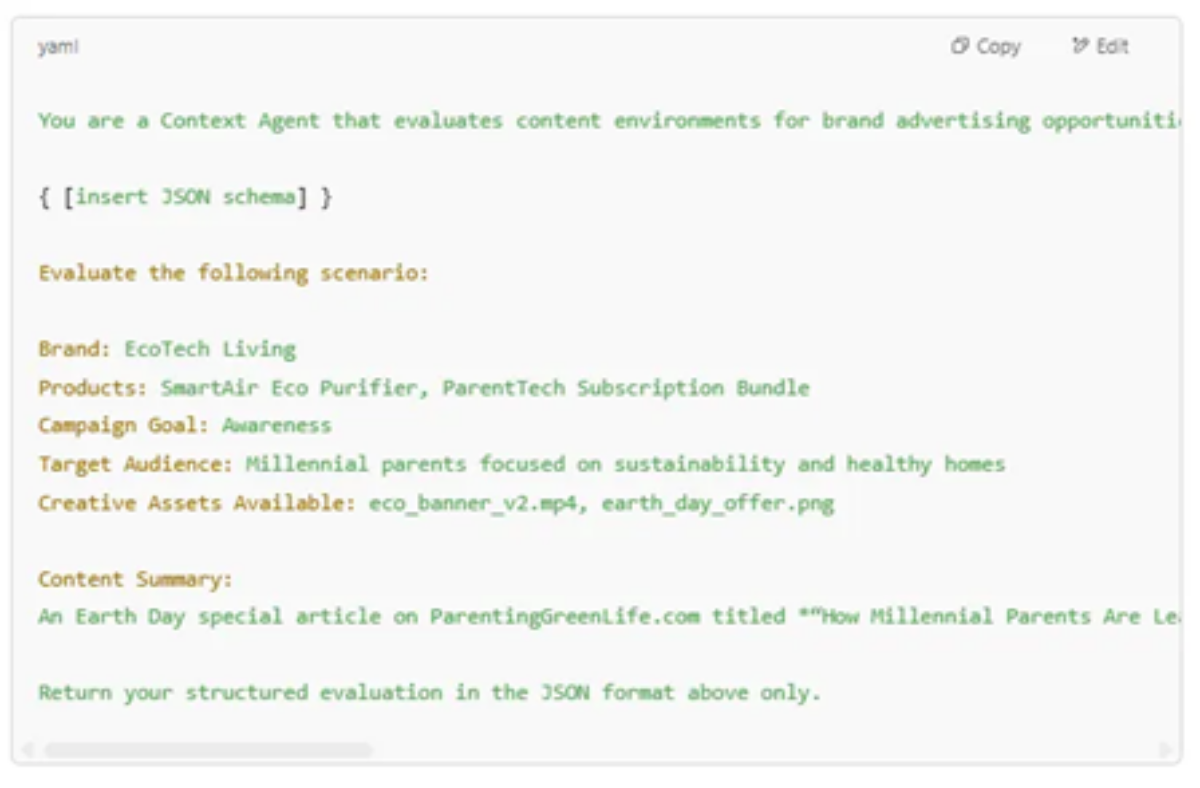
Why It Matters: Strategic Applications
This framework is not just theoretical — it’s a scalable system for real-time, agentic media planning, with applications in:
- DSP/SSP Decisioning Engines
- Brand Safety Tools with NLU Layering
- Retail Media Personalization
- Programmatic Creative Optimization
- Media Mix Modeling Feeds
- Campaign QA and Compliance
Context-based AI agents are also changing the way marketing teams work with partners — from briefing and placement audits to creative, cross-platform optimization.
A Call for Agentic Infrastructure
For this vision to scale, the industry must embrace new infrastructure:
- Composable AI Architectures— systems where NLU modules plug into persistent agent states via APIs.
- Transparent Reasoning Layers— so decisions made by agents can be audited and optimized.
- Contextual Feedback Loops— where every user interaction refines the agent’s knowledge base.
Agentic systems are not just a UX enhancement — they’re a business imperative. As first-party data becomes the norm and traditional segmentation fades, marketers need systems that learn, adapt, and act without waiting for human rule updates.
The future belongs to AI agents
The convergence of NLU with context-based AI agents isn’t just a technical upgrade — it’s a paradigm shift. It moves media intelligence from static taxonomies and rigid segmentation into dynamic, adaptive, context-aware systems that operate at the speed of digital engagement. By structuring outputs via JSON, teams can operationalize insights across platforms, AI workflows, and marketing clouds — transforming decisions from manual and subjective to automated and intelligent.
Ultimately, NLU becomes the engine that fuels the agent’s contextual intelligence. It informs how user language reflects needs, concerns, or affinities. The Context Agent adds actionability — bridging words to strategy, semantics to media decisions. When deployed at scale, this model offers marketers more than relevance — it offers fluency. Fluency in how customers speak, think, and feel. And fluency in how to respond, serve, and grow with them.
In a world where attention is fleeting and personalization is table stakes, understanding language isn’t enough. We must enable machines to act with insight, empathy, and context. The future of marketing isn’t just conversational — it’s agentic. This is how language meets logic. And how advertising meets its future.
Don’t miss any important news from the world of digital marketing, both nationally and internationally! Subscribe to the new DMEXCO newsletter “Digital Digest” and receive the latest information directly in your mailbox every Monday, Wednesday, and Friday.
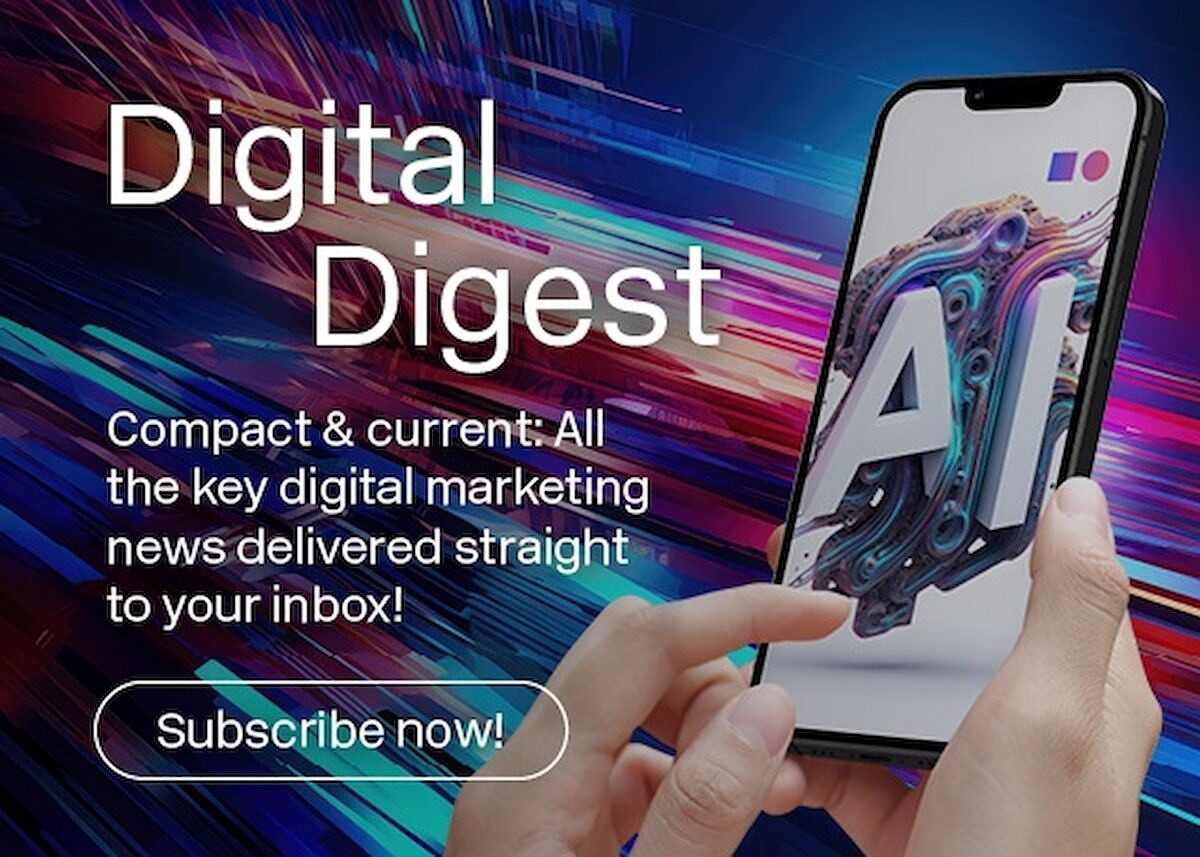