AI Ethics II: Why we need quality control
Artificial intelligence doesn't make mistakes, but that can be a big problem.
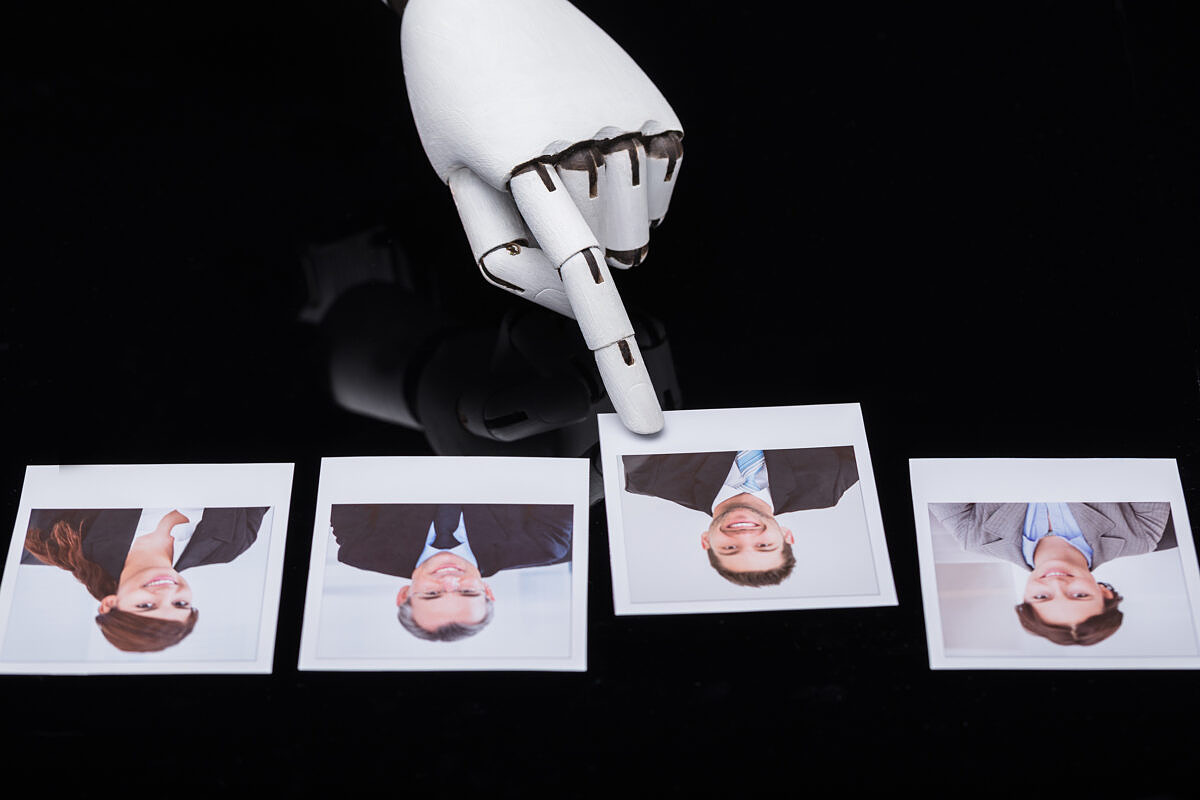
The first part of this little AI Ethics series already dealt with the issue of why we should not use artificial intelligence in all situations without hesitation. This second part shows why we also have to pay close attention even when AI is used for very commendable objectives.
Who controls the development of AI and ensures its social compatibility?
The question of what we really want to use AI for, such as monitoring the general population non-stop, will occupy us for many years to come. A completely different but by no means lesser issue consists of controlling AI. What exactly does it do, what data is used and how can it be ensured that the results correspond to societal values and standards?
The use of artificial intelligence always entails a certain loss of control. The various forms of AI—simple or complex algorithms, weak or a strong AI, machine or deep learning—are all largely nontransparent. We know that Facebook uses an algorithm to generate our personal news feed, but we can’t tell why certain content is displayed to us and certain content is withheld from us. Facebook doesn’t even know that. Only the algorithm does. Nevertheless, it is part of our personal reality.
How problematic the transfer of decisions to an AI can be is shown by various examples in which, paradoxically, the aim was to avoid discrimination and disadvantages. AI applications already support us in receiving loans, in selecting the best candidates for job interviews, or in calculating the probability of a criminal becoming a repeat offender. In all these areas there has been frequent discrimination in the past. AI is to be used to change this. This is based on the assumption that AI judges on a purely objective basis, because it is free of discriminatory or emotional thinking.
However, these artificial decision-makers must be trained with great effort so that they can meet the desired requirements. They are not intelligent in the sense that you can simply give them a new task and they will perform it as desired. They need as much data as possible, from which they can derive the required patterns and regularity. The data used for this purpose significantly determines the quality of the subsequent results and is at the same time often the weak point. Since it is supposed to simulate human decisions, such decisions from the past are usually also used for training. Under certain circumstances, the database may contain unwanted patterns that AI recognizes and uses. In the worst-case scenario, however, the discriminatory elements in the decisions increase when AI recognizes discrimination as a pattern. It also lacks the moral compass with which a person can reconsider their decisions. In addition, there is a lack of transparency in the database and a lack of awareness that AI decisions can also be discriminatory.
AI can discriminate, too
Confidence in the decisions made by artificial intelligence is increasing, as a Bitkom survey shows: “In certain situations, 6 out of 10 German citizens would rather accept a decision made by AI than that made by a human being”. But is this trust justified?
There are examples that show the opposite. As early as 2014, Amazon developed an application robot that would automatically pre-select the most suitable candidates for a vacant position from the large number of applications. As it turned out, AI put women at a disadvantage. The reason is pattern recognition that the developers did not foresee. Since more men than women work in the technology industry, AI concluded that men are more likely to be enthusiastic about the company and filtered out women. There are other very similar examples, in which incoming applications have been compared with successful recruitment in recent years. This is actually a sensible procedure, but there are often hidden discriminatory patterns in the data on new hires that AI recognizes and subsequently uses itself.
Harvard student Tyler Vigen shows with his “Spurious Correlations” that we do not foresee all patterns that are recognized by an algorithm. For example, there is a 99.79 percent correlation between US spending on science, space and technology and the number of suicides caused by hanging, strangulation and suffocation. At least that’s what the data indicates. We quickly suspect that this cannot be a cause-effect relationship. A machine, however, only understands the significance of the data, because a plausibility check requires a fundamental understanding of the objects under investigation and AI does not possess this capability.
Further examples
ProPublica lists numerous cases in which the data-based predictions of repeat offenses by criminals in the USA were marked by racist prejudices.
Researchers found that human language is not suitable for training AI, as cultural prejudices otherwise manifest themselves.
A very open training can also quickly cause problems, as Microsoft experienced with its chat bot Tay.
The bottom line:
The development of non-discriminatory AI depends on a data set that does not itself contain any discriminatory patterns. Recognizing this is the real challenge, because disadvantages are not always obvious. We must also remember that AI discrimination is not always unwanted and can even be used specifically to give the appearance of objectivity.
Outlook: In the third and final part of the AI Ethics series, we present various initiatives that have already formulated requirements for AI control.